Media companies attract data talent on limited budget
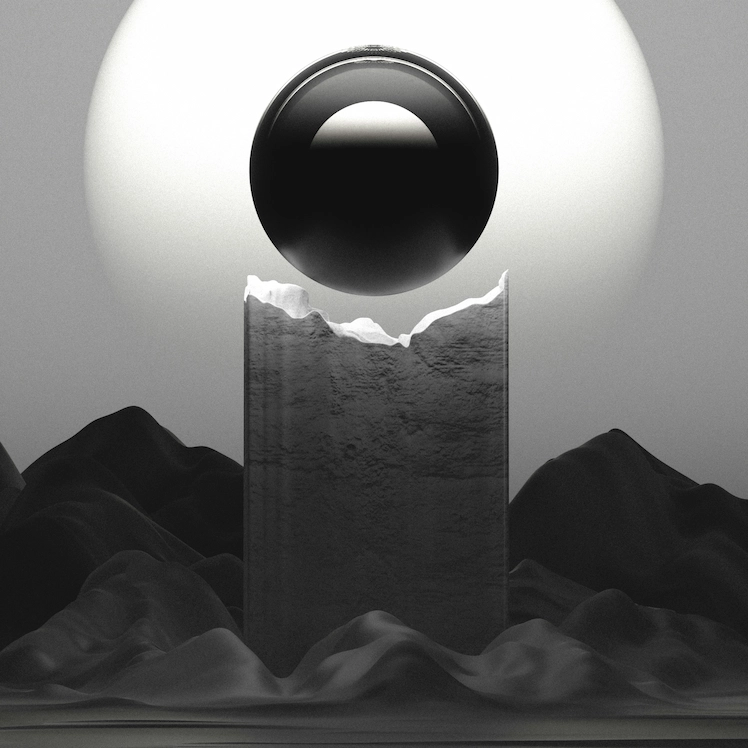
- Das ist eine H2
- Das ist eine H3
Data is the new gold - especially in the media industry. From personalized news feeds to optimized ad delivery to predicting content trends: Data competency is no longer a "nice-to-have" but a decisive competitive factor. But while the demand for data experts is rapidly increasing, media companies often face a particular challenge: How do you attract sought-after talent when budgets are tight and you're competing with financially powerful tech giants?
This chapter examines the specific requirements for data experts in the media world, analyzes the competitive disadvantages, and presents concrete solutions for how media companies can nevertheless become attractive employers for data talent.
1. Wanted: The "Media-Data Unicorn"? Requirements for Data Experts
Data experts in the media industry need more than just technical know-how. Beyond solid knowledge in statistics, machine learning, and data visualization, specific skills and a certain mindset are required:
- Domain knowledge: An understanding of the media landscape, content formats, user behavior, advertising markets, and editorial processes is essential. Data must be interpreted in context.
- Communication strength: Data experts must be able to communicate complex analyses and insights comprehensibly to different stakeholders (editors, marketing, management) - often in the form of data storytelling.
- Agility & curiosity: The media industry moves quickly. Experts must be ready to quickly familiarize themselves with new topics, work with incomplete data, and find creative solution paths.
- Ethics awareness: Handling user data requires a high degree of responsibility and knowledge of data protection regulations (GDPR, etc.).
The challenge lies in finding talent that brings both technical depth and this specific industry understanding along with the right soft skills.
2. The Hurdles: Competitive Disadvantages in the Talent Market
Media companies often face significant disadvantages in the "war for talent" for data experts:
- Salary gap: Tech companies, banks, or consulting firms can often pay significantly higher salaries. According to current surveys from the StepStone Salary Report, data scientists in media companies earn on average 15-20% less than in the IT industry and up to 30% less than in the financial sector. For many media houses, it's difficult to compete here.
- Technological infrastructure: Not rarely, media companies struggle with outdated systems or a fragmented data landscape. Modern, cloud-based analysis environments are not always standard, which complicates daily work and can cause frustration.
- Unclear career paths: The role of data experts is still relatively new in many media companies. Defined career paths and development opportunities are sometimes less clearly structured than in established tech corporations.
- Perception vs. reality: The image of some media companies may not be as "technology-driven" as that of pure digital companies, even if exciting data projects are running behind the scenes.
- Knowledge monopolies and lone fighters: Data competence in media companies often concentrates on a few "data experts" who act as lone fighters. This isolated position without like-minded colleagues and exchange opportunities deters many talents.
3. Solution Approaches: Creativity Beats (Pure) Capital
Despite the challenges, media companies can score points if they proceed strategically and creatively:
Building Data Literacy Throughout the Company
Goal: Not everyone needs to be a data scientist, but a basic understanding of data throughout the company raises the level and relieves specialists.
Measures: Internal training, workshops, e-learning offerings for editors, marketing, and sales staff. Promotion of a data-informed culture. This makes the company more attractive for experts who want to work in a data-affine environment.
Case study: The Axel Springer Academy has expanded its program and with its "Academy for Journalism and Technology" specifically trains young talent at the intersection of journalism and technology. The program includes basic modules on data analysis and visualization for all participants, specialized tracks for Data Journalism, and practical projects with real datasets. This approach has proven successful in attracting and retaining young talent with hybrid competence profiles.
Strategic Talent Acquisition and Retention
- Employer branding: Clearly communicate what exciting, unique data projects the media house offers (e.g., work with exclusive content data, analysis of millions of user interactions). Emphasize the "purpose" of the work in the media environment (social relevance, information mission).
- Focus on development: Even if the salary isn't always top-notch – clear development plans, training opportunities (conferences, certifications), mentoring programs, and the chance to quickly take on responsibility are strong arguments.
- Flexible working models: Home office, flexible working hours, and a good work-life balance are more important to many talents than the last percentage of salary.
Overcoming Knowledge Monopolies
A common problem in media companies is the concentration of data knowledge among individual employees. Strategies to overcome this:
- Establish a documentation culture: Systematically document processes, analyses, and insights so that knowledge remains in the company and is shared
- Introduce pair working: Working together on data analyses promotes knowledge transfer and breaks down silos
- Internal training by experts: Regular knowledge-sharing sessions make expert knowledge accessible to all
- Rotation programs: Temporary exchange of employees between data and specialist departments spreads data competence
Data Ethics as a Competitive Advantage
- Clear commitment: Transparent and ethically responsible handling of data is not only an obligation but can be actively communicated as an advantage. This attracts talent for whom values are important.
- Involvement: Give experts the opportunity to participate in shaping data policies and ethical standards.
Promoting Diversity, Achieving Better Results
Diverse teams bring different perspectives to data analysis and can thus lead to better results:
- Actively promote diversity: Specifically look for diverse talent and develop recruitment strategies for underrepresented groups
- Raise awareness of unconscious bias: Training for managers can help identify and overcome unconscious biases in recruiting
- Strengthen innovation power: Experience shows that diverse teams develop more innovative solution approaches – a real competitive advantage in interpreting complex user data
The media group Bertelsmann was able to increase the proportion of women in digital roles across the group through its "Diversity & Inclusion" program with targeted measures to promote women in technical professions. The "Digital Female Leadership" program, which promotes and networks young talent, was particularly successful. An internal study at Bertelsmann subsidiary Arvato Systems also showed that teams with higher diversity achieved measurably better results in terms of user-friendliness and target group appeal in the development of user analysis tools.
Practical example: How MediaPrint became a magnet for data talent
MediaPrint's Data Strategy Project provides a concrete example of a successful talent strategy: Through a clear vision, participatory development, and measurable success (80% increase in efficiency, 87% data quality score), the newspaper publisher managed to create an attractive environment for data experts.
4. Practical Tip: 7 Creative Ways to Attract and Retain Data Talent
Beyond the major strategies, there are concrete, often budget-friendly measures:
- Project showcases: Present exciting, completed data projects (anonymized) on career websites, in professional blogs, or at conferences. Show what's possible!
- Cooperation with universities: Offer internships, working student positions, or thesis projects. Build relationships with potential talent early on. Guest lectures at universities increase visibility.
- Focus on unique datasets: Emphasize access to exclusive media data (user behavior, content performance, market research) that tech companies don't have in the same way.
- "Brown bag sessions" / knowledge exchange: Regular, informal meetings where data experts (including external guests) present projects or discuss new tools. This promotes learning and community.
- Mentoring programs (also cross-functional): Couple experienced data experts with young talent or with employees from other departments (e.g., editorial) to promote understanding.
- Emphasis on impact & visibility: Show how the work of data experts directly contributes to the success of content, products, or user retention. Give them a stage for their successes.
Conclusion
Media companies can compete in the race for data talent, even if budgets are tighter. The key lies in specifically playing to their own strengths – unique data, social relevance, often more flexible structures – and creating a culture where data competence is valued and experts can develop.
It's also important to deliberately break down knowledge monopolies and anchor data competence more broadly in the company. With creativity, strategic focus, conscious promotion of diversity, and a clear commitment to data ethics, media houses can become true talent magnets.
Related Articles in our series on data-driven strategies for media companies
- Data as a Strategic Compass: Learn how to effectively use data as a navigation aid for strategic decisions. With practical tips and success stories from the media industry.
- Garbage In, Garbage Out: Why data quality and integration are the crucial foundations of any digital strategy – and how to ensure them in your media company.
- Data Protection as a Competitive Advantage: How to balance data protection and personalization to develop a strategic advantage for your publishing house or media company.
- Bridges Instead of Walls: How Media Companies Overcome Data Silos: This article shows how publishers and media companies can overcome their data silos and increase their competitiveness.
- Becoming a Talent Magnet: Successfully Attracting Data Talent: Examines requirements, competitive disadvantages, and presents concrete solutions for how media companies can become attractive employers for data talent.
- Data vs. Gut Feeling: The Cultural Shift in Newsrooms How media companies can successfully manage the cultural transition to data-driven decision-making.
- The Path to a Data-Driven Media Organization: A Roadmap: Findings from the series of articles in which various facets and challenges were examined.
Would you like to learn more about this topic? Subscribe to our newsletter to receive more articles from our data competence series. Contact us at contact@datainstitute.io or schedule an appointment for individual consultation on overcoming data silos in your media company.
Photo Wallsbysmit on Unsplash

Just subscribe
and receive monthly newsletters
Just subscribe
and receive monthly newsletters

Just subscribe
and receive monthly newsletters

Related case studies
There are suitable case studies on this topic
Which services fit this topic?


